Optical Character Recognition (OCR)
What is Optical Character Recognition (OCR)?
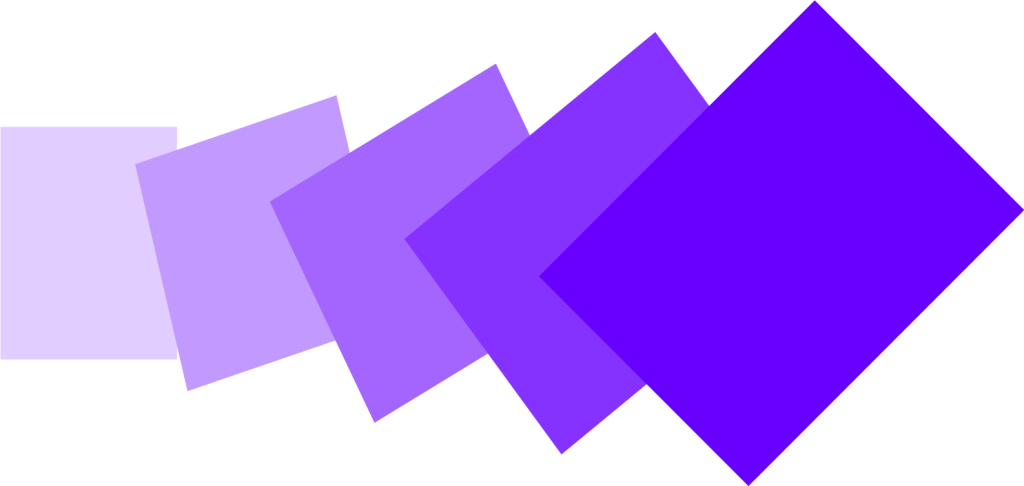
OCR software can reduce manual data entry by up to 70-80%
Optical Character Recognition (OCR) is a technology that makes it possible to automatically recognize printed or handwritten text in digital images or scanned documents and convert it into machine-readable text. OCR systems can extract text from various sources, such as PDFs, contracts, photos or handwritten notes.
OCR technology uses image processing and pattern recognition algorithms to analyze the shape and structure of letters, numbers and symbols in an image and convert them into digital text accordingly. Modern OCR systems are able to recognize a variety of fonts and styles and can even deal with distortion, different lighting conditions and background noise.
Four reasons for Optical Character Recognition (OCR)
Increased efficiency
Manual data entry and processing is time consuming and prone to human error. OCR enables companies to significantly speed up these processes and increase the accuracy of captured data, resulting in higher productivity and cost efficiency.
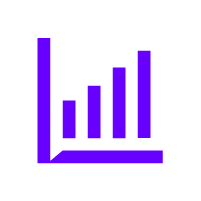
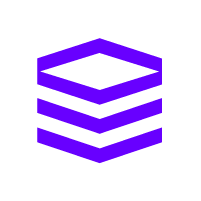
Automation of business processes
OCR technology can help automate business processes by enabling information to be automatically extracted from documents such as invoices, purchase orders and contracts and processed in business systems. This can help to reduce processing time and increase the overall speed of business processes.
Digitization and archiving
Many organizations have extensive archives of physical documents that are difficult to access and prone to damage or loss. OCR facilitates the digitization and archiving of these documents, making them more searchable, accessible and safer to store.
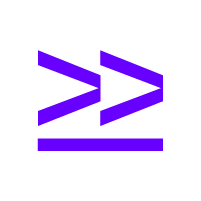
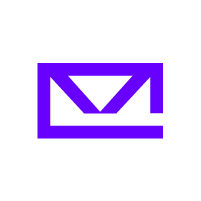
Integration of data from different sources
Companies often receive information from different sources and in different formats, such as emails, PDF files, scanned images or physical documents. OCR makes it possible to extract this data efficiently and consolidate it into a standardized digital format. This facilitates data analysis, the exchange of information between departments and decision-making by providing centralized access to all relevant data.
Four challenges for the use of OCR
Picture quality
The accuracy of OCR systems depends heavily on the quality of the scanned or digitized images. Blurred, distorted or poorly exposed images can lead to errors in text recognition.
Complex layouts and fonts
OCR systems may have difficulty extracting text from documents with complex layouts, multiple columns or unusual fonts. In such cases, text recognition may be less accurate.
Handwritten texts
Recognizing handwritten text is more difficult for OCR systems than printed text. Different handwriting, writing styles and irregularities can affect the accuracy of text recognition.
Integration into existing systems
Companies may need to adapt their existing IT infrastructures and business processes to effectively integrate OCR technology and reap the benefits of automated text recognition.
The importance of the training and learning process
The training and learning process of OCR software can have a significant impact on the results and performance of the system. Here are some aspects of how the training and learning process affects OCR results:
1. Improving accuracy
Well-trained OCR software can recognize text from images and scanned documents with greater accuracy. By using machine learning and artificial intelligence, the software can improve over time by learning from the input training data and adapting its recognition algorithms.
2. Recognition of different fonts and layouts
The training and learning process enables the OCR software to recognize a wider range of fonts, writing styles and layouts. The more diverse the training data is, the better the software can handle different text formats.
3. Handwriting recognition
OCR systems that have been specifically trained to recognize handwritten text can improve their performance in this area. By using training data that contains different handwriting and styles, the software can adapt its algorithms to better recognize handwritten text.
4. Adaptation to different languages
By training the OCR software with texts in different languages and writing systems, it can be better prepared to recognize texts in different languages and fonts. This enables the software to process multilingual documents more effectively.
5. Reduction of errors
The training and learning process can help to reduce the number of errors that occur during text recognition. The better trained the OCR software is, the less likely it is to recognize incorrect characters or overlook text elements.
Our technology partners
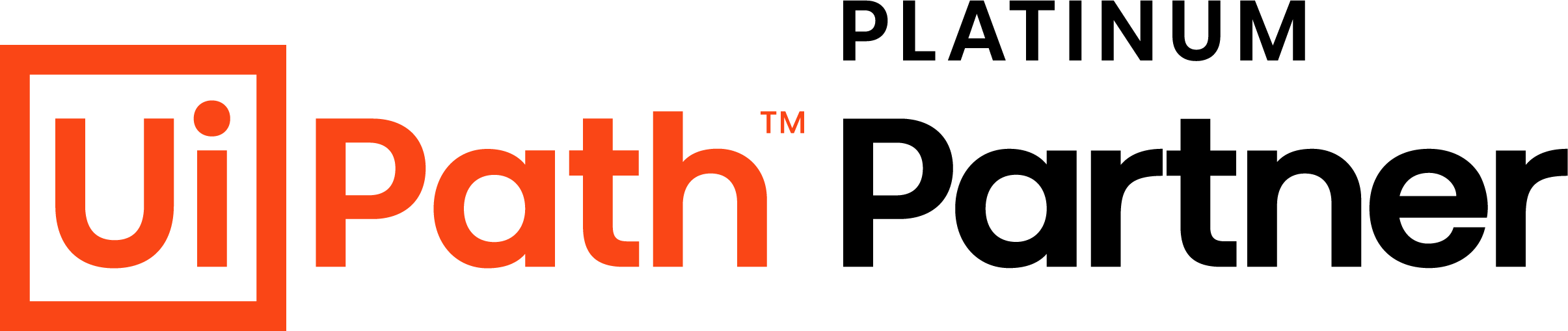
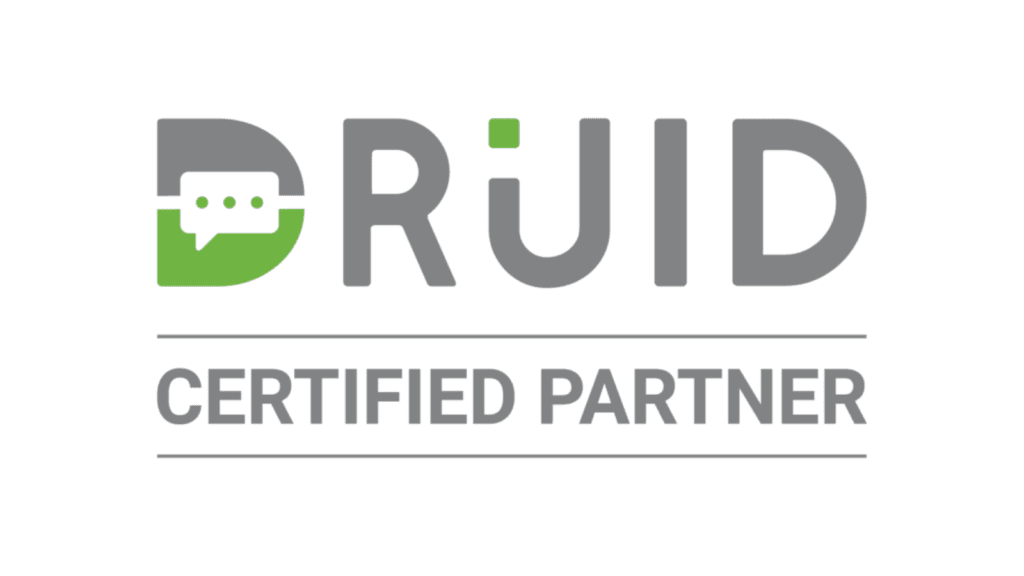
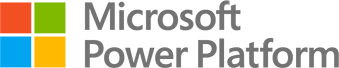
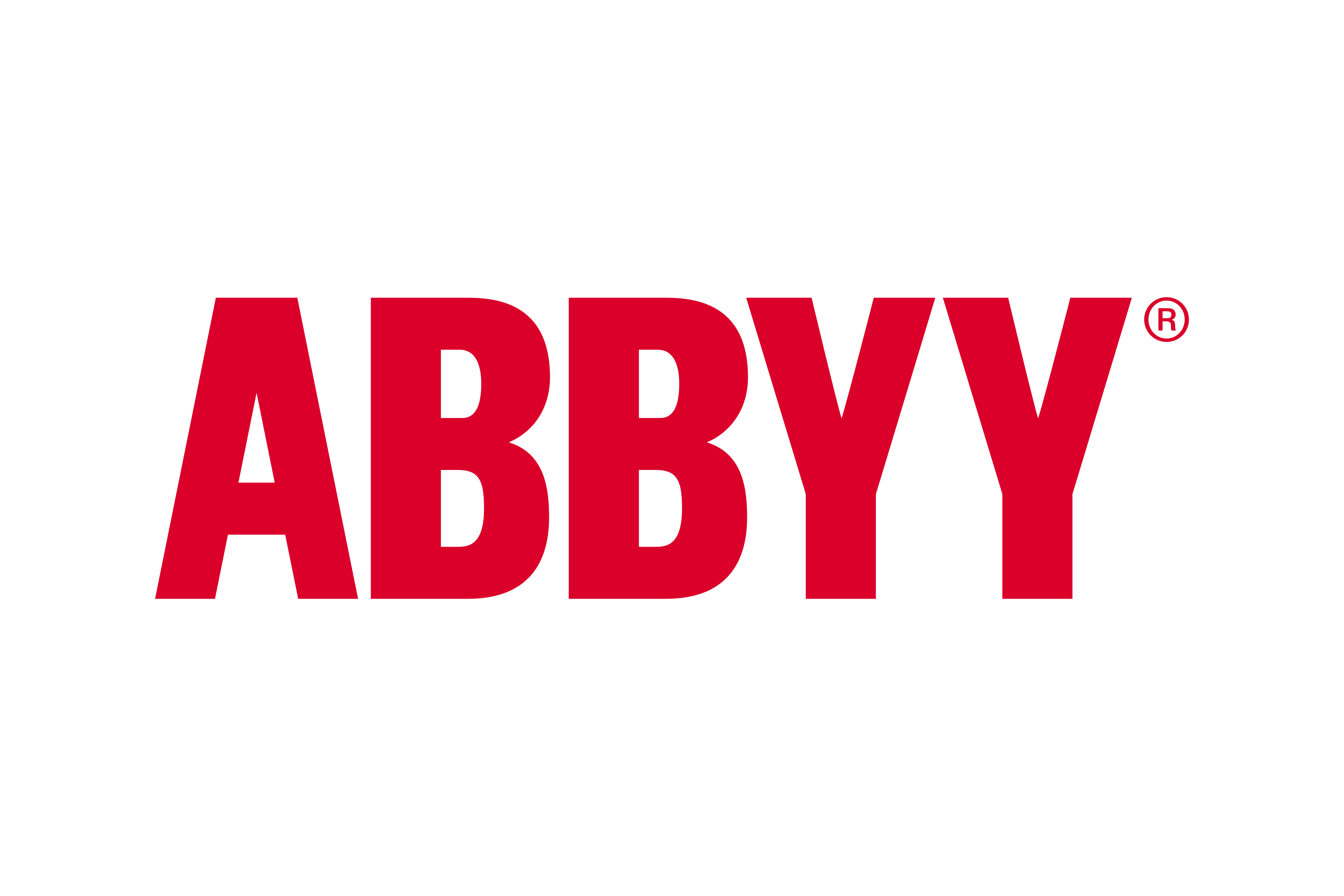