Human in the Loop in automation projects
Human in the Loop in automation projects
The reason why humans are indispensable for many automation projects
The reason why humans are indispensable for many automation projects
Artificial Intelligence (AI) is changing the world of work. But automation will not make human intelligence superfluous. For the vast majority of all automation projects, artificial intelligence relies on human help to play to its strengths. We explain what you need to know about human-in-the-loop models.
What does Human in the Loop mean?
Artificial intelligence aims to automate intelligent actions. Tasks that are normally performed by humans are completed by an AI. This opens up great potential for the automation of data-intensive tasks in all sectors and all areas of a company. In many cases, however, it is not efficient to completely remove humans from the process. Instead, human-in-the-loop combines human and artificial intelligence in an integrated process.
Humans learn through experience, an AI learns through data. Supervised learning uses qualified data sets that have been specially prepared to train the algorithm. By “labeling” the input with the respective output, the AI can derive patterns and apply them to the new data sets.
The model is then fed with the data. Supervised learning is used in two areas: In classification, an algorithm sorts data (e.g. in texts or images) into predefined classes based on patterns and similarities. In regression, an algorithm estimates the quantitative extent of a target variable based on the relationships between different variables in the data. Regression is used where figures need to be predicted – for example in sales forecasting.
In the next step, people adapt the machine learning model to avoid typical problems, such as overfitting, and to integrate important special cases into the model. In particular, cases with a lower level of certainty are checked manually and the result of the check is fed back to the model.
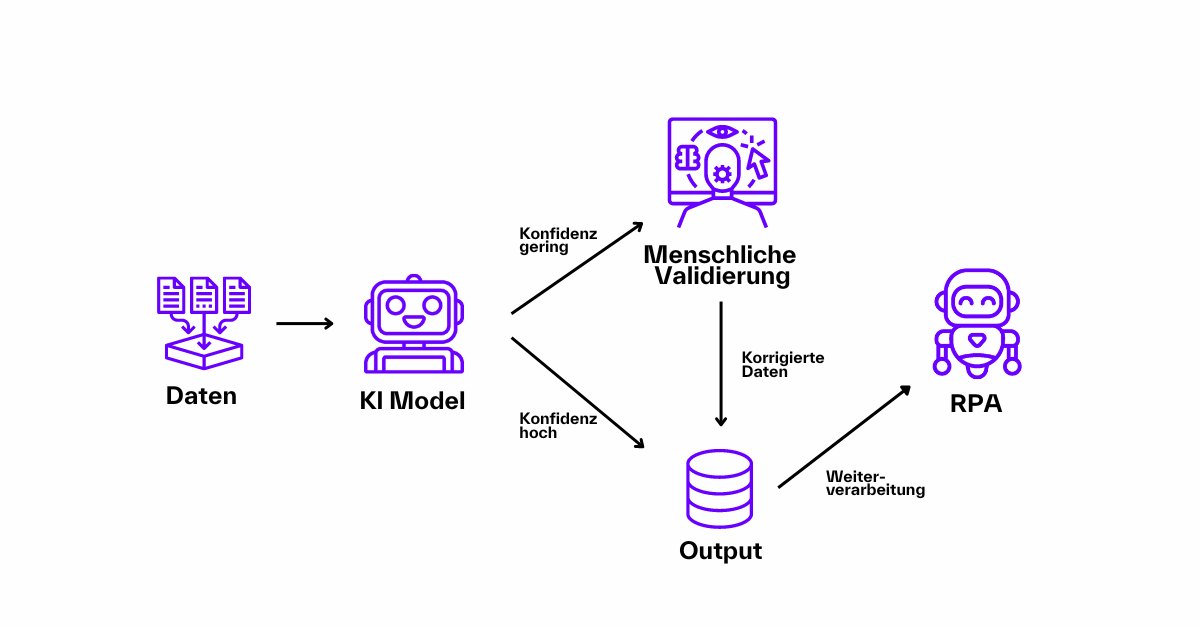
Human in the Loop: Advantages and use cases
Higher accuracy
Artificial intelligence can never achieve 100% accuracy. The technology is based on statistical methods that only know probabilities. Humans can often make statements with greater certainty. In many cases, this combination can systematically produce better results than a machine-only approach.
Making specialist knowledge usable
By integrating specific empirical knowledge, the algorithm’s blind spots can be illuminated. For example, NASDAQ uses a human-in-the-loop approach with its tool for monitoring trading activities on the stock exchange, in which experts pass on their knowledge to the algorithm via feedback loops.
Borderline cases
Large amounts of data are not publicly available for every problem. For example, if a chatbot is to understand user input in a rare language or a local dialect, the algorithm is dependent on human feedback. Facebook uses a human-in-the-loop model to analyse communication on the social media platform because not every aspect of human communication can be extrapolated from the data.
Recognize bias
The problem of hidden biases is primarily known from behavioral research. Even data sets are not neutral in every respect, but can contain unrecognized but systematic dependencies on influencing factors that have not been taken into account. Just as the way in which a question is asked can influence the answers, the way in which the data is collected can influence how the AI interprets the data. If it comes from public sources, the way the data is obtained may not be fully traceable. Without human intervention, an algorithm would take the bias from the data. A human-in-the-loop can often identify any bias before it becomes entrenched in the algorithm.
Areas of application for AI
AI is being used to accelerate innovation in areas ranging from everyday life to business intelligence. Here are the 5 most important application areas.
Forecasting & Time Series
Applying AI to forecasting and time series analysis allows us to better uncover the patterns hidden in data - trends, seasonality, irregularities/noise and cyclicality. Time series analysis can be useful to see how a particular asset, security or economic variable changes over time. It can also be used to examine changes between variables over the same time period. This makes it possible to predict future developments. Prediction by AI has proven to be an effective method for detecting patterns in both structured and unstructured data and predicting their future course.
OCR & Computer Vision
Computer vision is a function that is primarily concerned with analyzing the user interface. It is used to structure and identify individual elements of the displayed surface. OCR (Optical Character Recognition) is a subtype of computer vision. With OCR, characters can be extracted from images and any surfaces whose texts are not digitally tangible and made available digitally. It does not matter in which font or language the characters are displayed. OCR technology is already widespread and is used in the digitization of texts in invoices, receipts and other documents that are available as scans or photos.
Insights & Analytics
AI encompasses a variety of technologies for extracting insights and patterns from big data and deriving predictions based on this information. This includes analytics data from sources such as Google Analytics, automation platforms, content management systems, CRMs and more. AI analytics refers to a subset of business intelligence (BI) where software in the process of analyzing data exhibits behaviors normally attributed to humans, such as learning and reasoning. In practice, this means that AI automates the steps that a human would take to perform a comprehensive analysis of big data.
NLP & Chatbots
NLP (Natural Language Processing) is used to extract information from natural or human language and is therefore used in all areas of process automation in which free texts need to be analyzed and data extracted. For example, NLP can be used to recognize invoice numbers, amounts and data from invoice texts, regardless of their structure. NLP also enables the creation of chatbots that can be embedded and used in all common messaging applications.
Fraud & Anomaly Detection
Anomaly detection (or outlier detection) is the identification of rare elements, events or observations that arouse suspicion because they differ significantly from the majority of the data. Fraud detection is a typical example of anomaly detection. AI helps to complete the data analysis within milliseconds and also to recognize subtle and complex patterns that are difficult for the fraud analyst to detect. AI relieves employees of time-consuming tasks and allows them to focus on critical cases, e.g. when the risk values are at their highest.
Gain insights
Discover how AI can be used in your company.
Human in the Loop in practice: Classification of customer data
How human-in-the-loop can lead to better automated processes is demonstrated by the automation of verification processes in the context of legally prescribed know-your-customer policies, where (partial) automation is essential. For example, an intelligent algorithm can compare and validate uploaded selfies with the photo of the ID document. Let’s assume an algorithm is to compare a selfie photo with the photo of an ID card. This application requires a high level of accuracy that AI alone cannot achieve:
- In the first step, employees create the data sets with which the algorithm is trained. In the example, employees would create sets of selfie and ID document photos and assign a corresponding value (correct / incorrect) to each. The algorithm is fed with this training data and learns to recognize features based on which it can correctly classify the image pairs. The algorithm outputs one of these values for each set. A human then checks the algorithm’s output and feeds it this result in turn. This creates a feedback loop – and the algorithm gets better and better at classifying the data.
- There is always data for which the algorithm cannot provide a clear result. For example, there is not enough initial data available for ID documents from individual countries for the AI to be able to evaluate them effectively. If these cases fall below a confidence threshold, they are automatically forwarded to the employees to be checked manually and thus feed the algorithm with further valuable information.
In this model, the human is systematically integrated into the AI’s cycle, which systematically produces better results and learns faster than a model without a human in the feedback loop.
AI and RPA use case: Automatic processing of order confirmations
Find out in this use case how we helped our customer overcome their supplier management challenges using a combination of RPA and OCR.
- Industry: Manufacturing
- Department: Purchasing, supplier management
- System: ERP system, Office applications
- Technology used: RPA, UiPath Document Understanding (OCR)
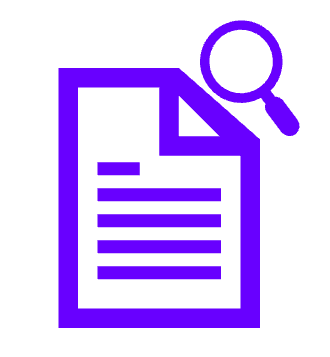
Conclusion: no AI without humans
Artificial intelligence will play an increasingly important role in companies in the future. However, this will not make humans superfluous – on the contrary: the preparation and processing of data will be essential for the successful development of intelligent algorithms. It is only through the systematic integration of human feedback that numerous applications can be realized with artificial intelligence: from predictive analytics to the automation of customer-related processes.